Artificial Intelligence (AI) is rapidly transforming industries and reshaping society. From self-driving cars to medical diagnostics and customer service chatbots, AI is making significant advancements that offer immense benefits. However, as AI systems become increasingly embedded in our daily lives, the ethical implications surrounding their development and use are becoming more pronounced. The potential for AI to impact human lives raises important questions about fairness, privacy, and accountability. In this article, we examine the ethical dilemmas surrounding AI, focusing on issues such as bias in algorithms, data privacy concerns, and the potential for job displacement.
1. Bias in Algorithms: Ensuring Fairness and Accountability
One of the most significant ethical challenges in AI is the potential for bias in the algorithms that power these systems. AI algorithms are often trained on large datasets, and if those datasets contain biased or unrepresentative information, the resulting AI systems may perpetuate those biases. This can have serious consequences, particularly when AI is used in sensitive areas like hiring, criminal justice, or lending.
Key Issues with Bias in AI:
- Data Bias: AI systems rely on data to make decisions, and if the data used to train an algorithm is skewed or incomplete, the AI may produce biased outcomes. For example, if an AI system used for hiring is trained primarily on resumes from a certain demographic, it may favor candidates from that group, unintentionally discriminating against others.
- Algorithmic Bias: Even if the data is unbiased, the way the algorithm is designed and trained can introduce bias. For instance, facial recognition systems have been shown to have higher error rates for people with darker skin tones, which can lead to unfair outcomes in security or surveillance contexts.
- Discrimination and Inequality: The use of biased AI systems can exacerbate existing social inequalities. For example, biased algorithms in the criminal justice system may lead to unfair sentencing or parole decisions, disproportionately affecting marginalized groups.
Examples in Action:
- COMPAS (Correctional Offender Management Profiling for Alternative Sanctions): A widely used risk assessment tool in the U.S. criminal justice system, COMPAS has faced criticism for being biased against Black defendants. The algorithm used in COMPAS was found to predict higher recidivism rates for Black offenders compared to white offenders, even when controlling for other factors.
- Amazon Recruitment Tool: Amazon scrapped its AI-driven recruitment tool after discovering it was biased against women. The tool was designed to help the company identify top candidates by analyzing resumes, but it was trained on resumes submitted to Amazon over the past decade, which were predominantly from men. As a result, the tool favored male candidates over female ones.
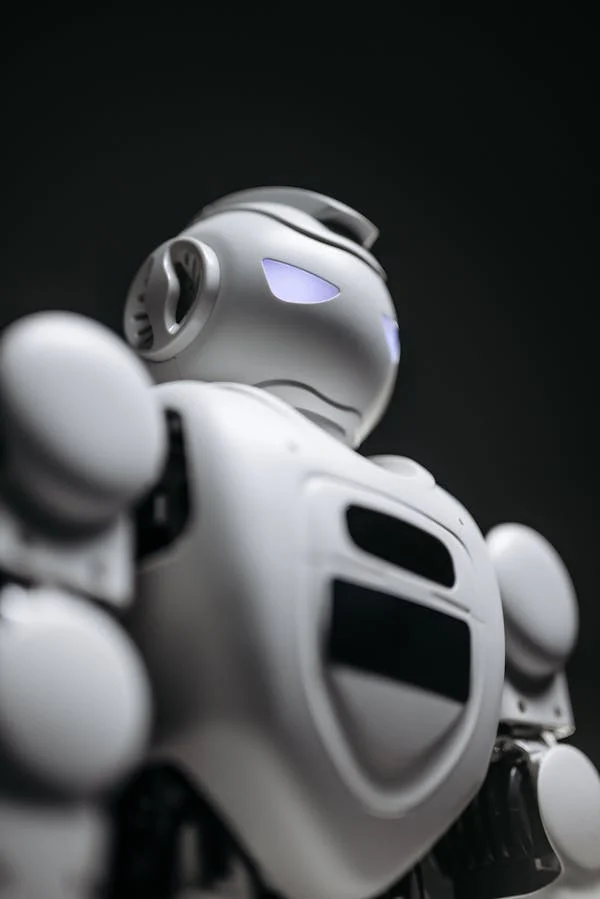
Solutions:
To address algorithmic bias, AI developers must ensure that the datasets used to train models are diverse, representative, and inclusive. Additionally, adopting fairness-aware machine learning techniques and conducting regular audits of AI systems can help identify and mitigate biases. Implementing transparency and accountability in AI decision-making is also essential to ensure that AI systems are fair and just.
2. Data Privacy Concerns: Protecting Personal Information
AI systems often rely on vast amounts of data to function effectively, and much of this data is personal in nature. Whether it’s health data, financial information, or browsing behavior, the data that powers AI systems can be highly sensitive. As AI continues to integrate into industries like healthcare, finance, and advertising, the ethical implications of data collection and use are becoming more critical.
Key Issues with Data Privacy:
- Informed Consent: Many AI systems collect data from individuals without their full understanding of how their data will be used. People often give consent to data collection without knowing the full extent of how their information might be utilized, whether it’s for targeted advertising, product recommendations, or other purposes.
- Data Security: The more data AI systems process, the greater the risk of data breaches and unauthorized access. If sensitive data is exposed, it can lead to identity theft, financial fraud, or violations of privacy rights. Securing data from cyberattacks and ensuring robust encryption methods are essential to protect personal information.
- Surveillance and Tracking: AI-powered surveillance systems, such as facial recognition and location tracking, raise significant concerns about personal privacy and civil liberties. Governments and corporations could use AI to track individuals’ movements, behaviors, and activities, potentially infringing on personal freedoms.
Examples in Action:
- Cambridge Analytica Scandal: The Cambridge Analytica scandal revealed how Facebook allowed third-party apps to harvest personal data from millions of users without their consent. This data was then used to target political advertisements, raising concerns about privacy violations and the manipulation of public opinion.
- Facial Recognition Technology: The widespread use of facial recognition technology, particularly in public spaces, has sparked debates about privacy. Companies like Clearview AI have faced backlash for scraping publicly available photos from social media to build facial recognition databases without users’ knowledge or consent.
Solutions:
To protect data privacy, AI developers and organizations must implement strict data protection policies, including anonymization, encryption, and secure storage. Clear and transparent data usage policies should be in place, ensuring that individuals are informed about how their data will be used and have the ability to opt-out. Regulatory frameworks like the General Data Protection Regulation (GDPR) in the European Union offer important guidelines for protecting personal data and holding organizations accountable for data misuse.
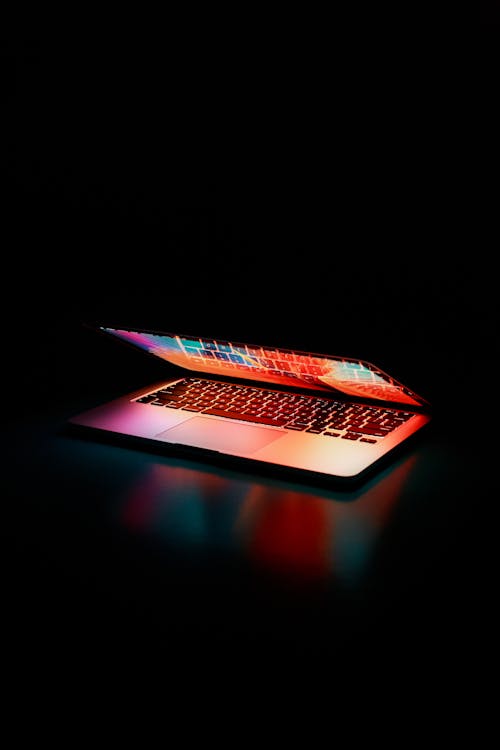
3. Job Displacement: Addressing the Impact on Employment
The rise of AI and automation has raised concerns about job displacement and the future of work. As AI systems become more capable of performing tasks traditionally done by humans, there is a growing fear that automation will lead to widespread job losses, particularly in sectors like manufacturing, retail, and transportation.
Key Issues with Job Displacement:
- Automation of Routine Tasks: Many jobs that involve repetitive, manual tasks are highly susceptible to automation. For example, AI-powered robots are increasingly being used in factories to assemble products, and autonomous vehicles are threatening jobs in trucking and delivery services.
- Shifting Job Roles: While AI may eliminate some jobs, it also has the potential to create new roles. However, these new roles often require advanced technical skills, which may not be accessible to workers in displaced industries. This raises concerns about the future of employment for lower-skilled workers.
- Economic Inequality: Job displacement caused by AI could exacerbate income inequality, as workers in industries vulnerable to automation may struggle to find new employment opportunities. The benefits of AI may be disproportionately enjoyed by those with the skills and resources to adapt to the new economy, while others may be left behind.
Examples in Action:
- Autonomous Trucks: Companies like Waymo and Tesla are developing self-driving trucks that could revolutionize the transportation industry. While this could lead to significant cost savings for companies, it also threatens millions of truck driving jobs in the U.S. alone.
- Retail Automation: Companies like Amazon are using AI-powered robots to automate tasks in warehouses, reducing the need for human workers. While this boosts efficiency, it also leads to job displacement for workers in low-skilled positions.
Solutions:
To address job displacement, governments, businesses, and educational institutions must invest in retraining and reskilling programs for workers affected by automation. Providing access to education in fields like AI, data science, and robotics can help workers transition to new roles. Additionally, policies such as universal basic income (UBI) or other social safety nets may be necessary to ensure that displaced workers have a means of support as they transition to new jobs.
4. Accountability and Transparency: Who Is Responsible for AI Decisions?
As AI systems become more autonomous, determining accountability for their actions becomes more complex. Who is responsible if an AI system makes a mistake or causes harm? Is it the developer, the company that deployed the AI, or the AI itself? Ensuring transparency in AI decision-making is essential to building trust and holding entities accountable for the impact of AI systems.
Key Issues with Accountability:
- Lack of Transparency: Many AI systems, especially those powered by deep learning algorithms, operate as “black boxes,” meaning their decision-making processes are not easily understood by humans. This lack of transparency makes it difficult to determine how decisions are made and whether they are ethical or fair.
- Legal Responsibility: In the case of accidents or harm caused by AI, determining liability is challenging. For example, if an autonomous vehicle causes an accident, who is legally responsible—the manufacturer, the software developer, or the vehicle owner?
Solutions:
To ensure accountability, AI developers should implement transparent design practices, making AI decision-making processes more explainable and understandable. Clear legal frameworks must be developed to assign responsibility for the actions of AI systems, ensuring that accountability is maintained at every level of AI deployment.
Conclusion
As AI continues to advance, the ethical dilemmas surrounding its development and deployment will become more pressing. Bias in algorithms, data privacy concerns, and job displacement are just a few of the critical challenges that must be addressed. By prioritizing fairness, transparency, and accountability, we can harness the power of AI while ensuring that it benefits society as a whole. Collaborative efforts between AI developers, policymakers, ethicists, and the public are essential to creating an ethical framework for AI that balances innovation with respect for human rights and social justice.
Leave a Reply